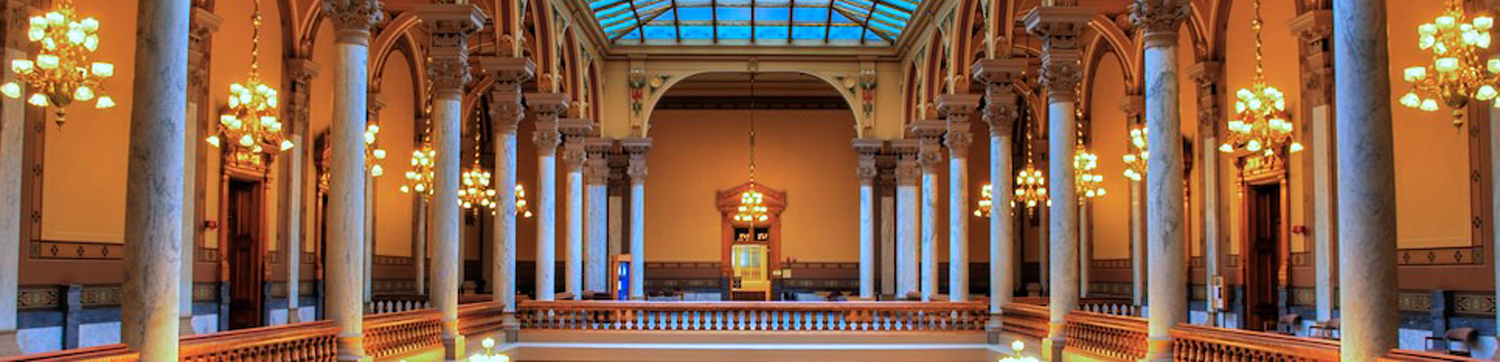
Abstract
As a fundamental concept of customer relationship management, customer lifetime value (CLV) serves as a crucial metric to identify profitable retail customers. Various methods are available to predict CLV in different contexts. With the development of consumer big data, modern statistics and machine learning algorithms have been gradually adopted in CLV modeling. We introduce two machine learning algorithms—the gradient boosting decision tree (GBDT) and the random forest (RF)—in retail customer CLV modeling and compare their predictive performance with two classical models—the Pareto/NBD (HB) and the Pareto/GGG. To ensure CLV prediction and customer identification robustness, we combined the predictions of the four models to determine which customers are the most—or least—profitable. Using 43 weeks of customer transaction data from a large retailer in China, we predicted customer value in the future 20 weeks. The results show that the predictive performance of GBDT and RF is generally better than that of the Pareto/NBD (HB) and Pareto/GGG models. Because the predictions are not entirely consistent, we combine them to identify profitable and unprofitable customers.
Recommended Citation
Sun, Yinglu; Cheng, Dong; Bandyopadhyay, Subir; and Xue, Wei
(2021)
"Profitable Retail Customer Identification Based on a Combined Prediction Strategy of Customer Lifetime Value,"
Midwest Social Sciences Journal: Vol. 24:
Iss.
1, Article 10.
DOI: https://doi.org/10.22543/0796.241.1053
Available at:
https://scholar.valpo.edu/mssj/vol24/iss1/10
Included in
Anthropology Commons, Business Commons, Criminology Commons, Economics Commons, Environmental Studies Commons, Gender and Sexuality Commons, Geography Commons, History Commons, International and Area Studies Commons, Political Science Commons, Psychology Commons, Urban Studies and Planning Commons